Key takeaways
- When more businesses join the Instawork platform, network effects bring benefits to existing partners as well.
- These benefits include higher fill rates, higher-quality workers, and higher satisfaction with completed shifts.
- The benefits are connected to the diversification of our business partners and not just higher volumes of shifts on our platform.
In a tight labor market like the one we're in now, competition for workers can be fierce. This is especially true for in-person hourly work, where the costs of switching between employers may be relatively small. So it's not surprising if some businesses want to keep staffing platforms like Instawork to themselves – and yet, our research shows that outcomes improve for everyone when our network of businesses expands.
We're not just talking about businesses booking more shifts on our platform. We're actually talking about more businesses booking shifts on our platform. The bigger our network of partners, the better outcomes we see, in markets all across the United States and Canada.
Why might this be the case? A lot of it has to do with our ability to match businesses with great workers. When more businesses join our platform, they bring along some of the workers who are already aware of them. But every business that joins also increases the chance that more highly skilled professionals will sign up on our platform to look for flexible work.
This is because the value of our platform has gone up for every worker – there are more offers of work for all of them to consider. It's the same principle that makes e-commerce websites like Amazon and eBay so attractive to buyers and sellers alike; by offering more options, they create a critical mass that pulls more people in.
Measuring the relationships
Identifying the benefits of expanding our network can take quite a bit of statistical heavy lifting. There's a technical note below that goes through most of the details. Here are the basics.
Each month, in every one of our markets, we can measure the concentration of businesses booking shifts using a metric called the Herfindahl-Hirschman Index. Then we can measure the relationship between the HHI and various outcomes of flexible work.
We're looking for how changes in shift outcomes relate to changes in the HHI at the regional level by month. The outcomes – all averaged for each month and region – are as follows:
- fill rate
- quality score achieved by workers prior to starting shifts (based on reliability, reviews, etc.)
- rating by businesses after shifts are completed
As we've launched recently in many new markets, here we're only including months where a market had 1,000 or more shifts. But you can see how these dynamics develop in a flexible labor market that's increasingly mature, like the Atlanta area. This is how the HHI tracked versus the share of shifts filled:
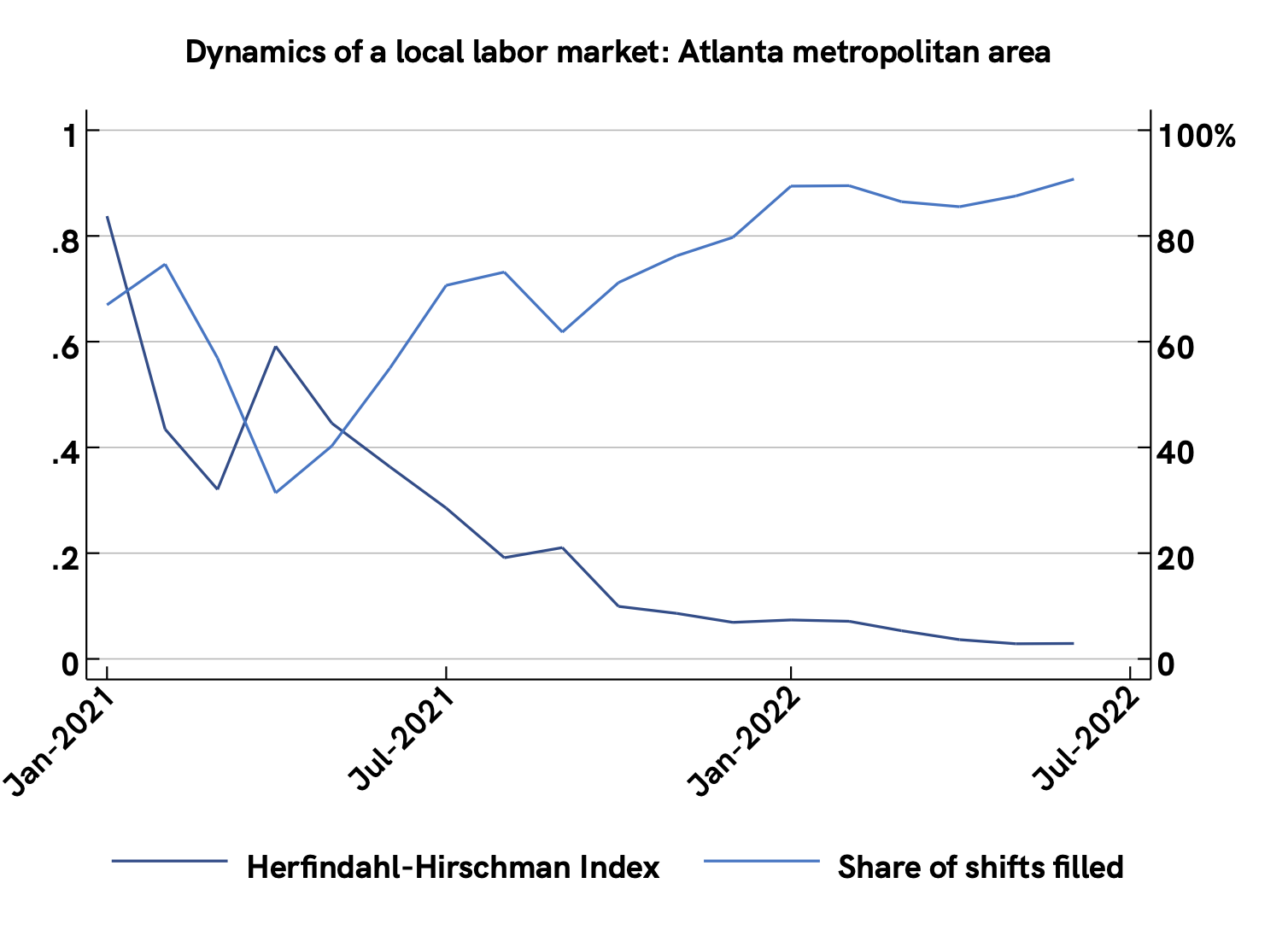
After some early bumpiness, when the market was relatively new on our platform, the share of filled shifts rose steadily as the HHI dropped. We see a similar trend for the worker score:
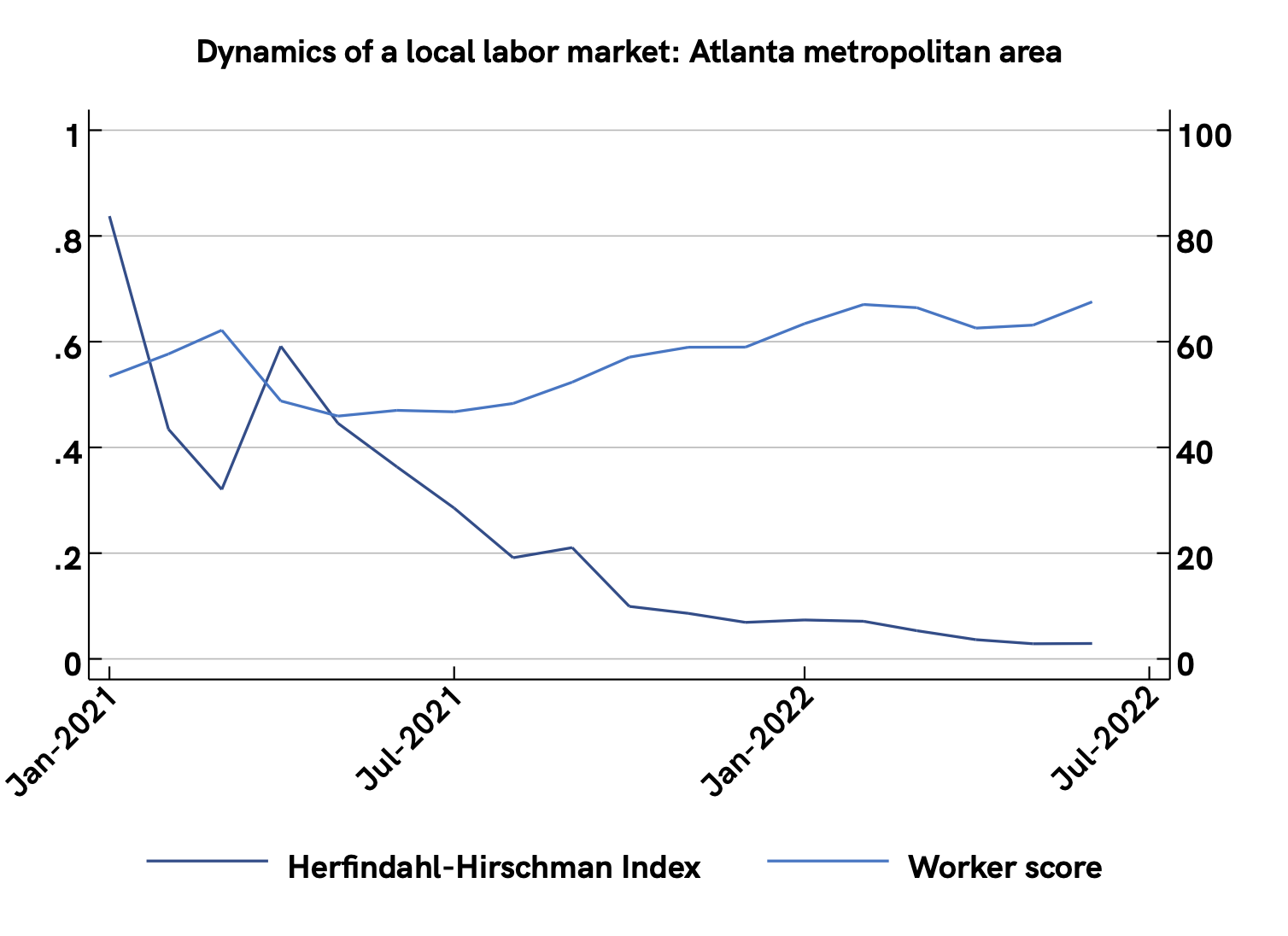
The data for ratings by businesses are somewhat lumpier, since businesses may not always rate shifts for workers whom they've rated before. But the trend is the same:
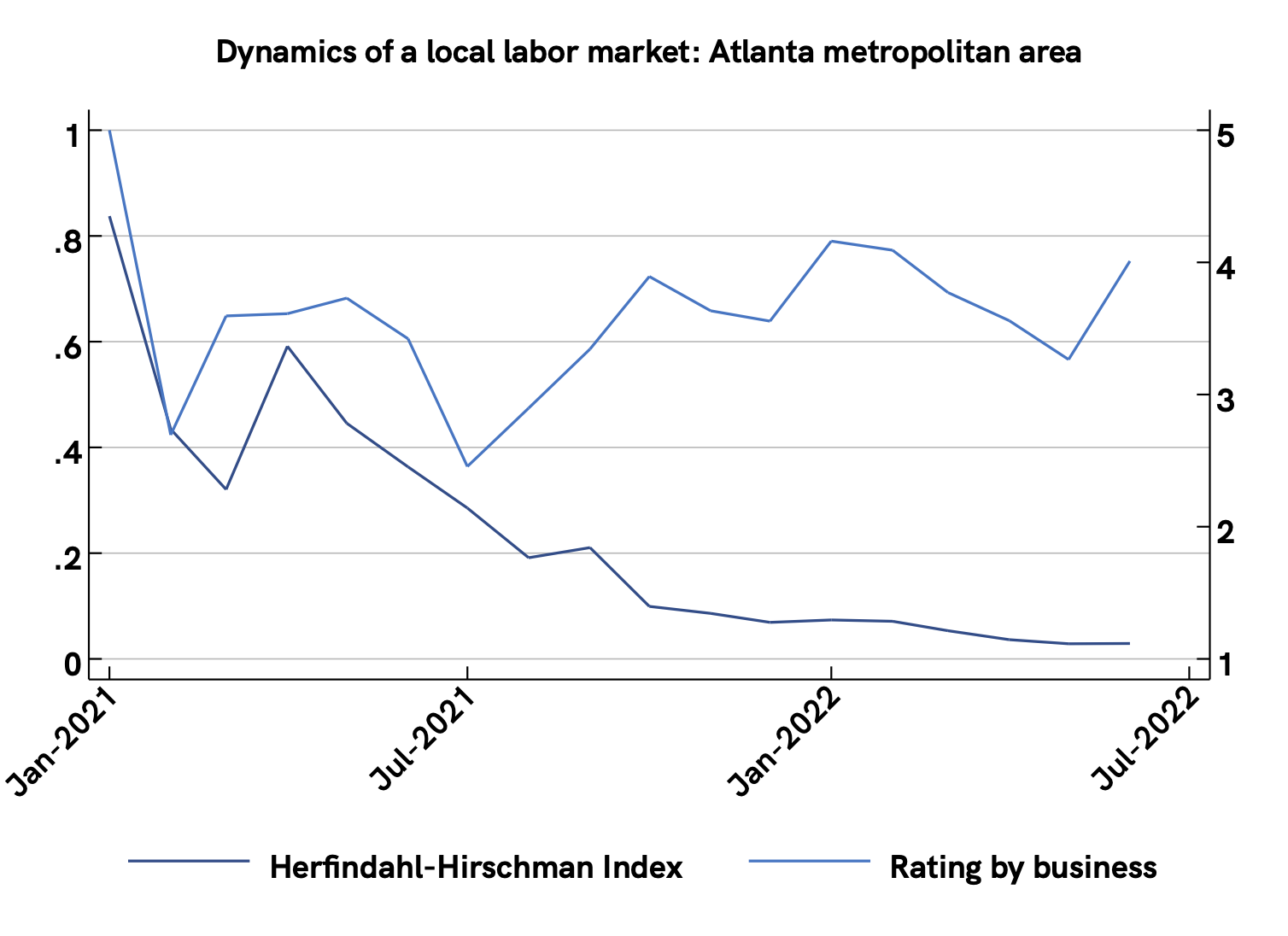
Now, here are the statistical estimates for our platform as a whole:
Average outcome
Scale for outcome
Effect of 0.1 point decrease in HHI
p-value
Fill rate0% to 100%+4.8%0.00Worker score0 to 100 points+1.8 points0.00Business rating 0 to 5+0.0380.02
We can see that lower concentration – a decrease in the HHI – is associated with higher fill rates, and also with higher worker scores. There may also be a very small positive effect on businesses' satisfaction with completed shifts. But overall, it seems like the most important relationship is between concentration and fill rate.
Reliable benefits
Can we count on seeing these improved outcomes whenever more businesses join our platform? Well, every market is different, and the statistical estimates above are based on data aggregated across our business. But these relationships look very strong indeed.
The bigger caveat is the possibility that the causality might work in the opposite direction. In other words, is it possible that, for example, higher fill rates actually cause concentration to fall, rather than the other way around? We might hypothesize that if fill rates went up, word would get around, and more businesses would want to use our services.
If that were the case, though, then we'd expect to see a positive relationship between shift volume (one of our controls) and our outcomes. Yet we don't. In all of our estimates, that relationship is either nonexistent or negative and of negligible importance. There's no sure thing in the labor market, but we think the growth of our network of partners is good news for every business on our platform.
[Technical note: The Herfindahl-Hirschman Index adds up the squared shares of all the businesses in the total shifts for the given month and region. So if only two businesses book shifts, and one books three quarters of them, then the HHI is 0.752 + 0.252 = 0.625. Higher values of the HHI indicate more concentration, while lower value indicate a more diverse pool of businesses. The HHI is always between zero and one.
To measure the relationships between the HHI and shift outcomes, we need to make a few adjustments. First, since the total number of shifts often has to increase in order for concentration to fall, we need to control for the total number of shifts in each month and region. Also, because we're tracking dozens of regions over time, we need to take account of the possibility that the regions are different from each other in some ways that don't change over time.
When we look at fill rate, worker quality, and post-shift ratings, we also need to control for changes in hourly pay. Higher pay makes shifts more attractive, so it makes filling shifts with great workers more likely. Finally, we have to recognize that our outcomes have upper and lower bounds. All of this leads us to a panel Tobit regression.]
Realtime metrics
These metrics, derived from data aggregated across the Instawork platform, compare the two weeks starting 6/16/2022 to the previous two weeks. To control for the overall growth of the Instawork marketplace, only shifts involving businesses that booked shifts in both periods are included:
- $0.04 drop in hourly pay
- 0.5% point drop in share of short-notice shifts
- 1.3 hours rise in hours per existing worker
To receive future briefings and data insights from our Economic Research team, please subscribe below. Follow Daniel Altman on Twitter at @AltmanEcon or on LinkedIn.